No-UI: How to Build Transparent Interaction
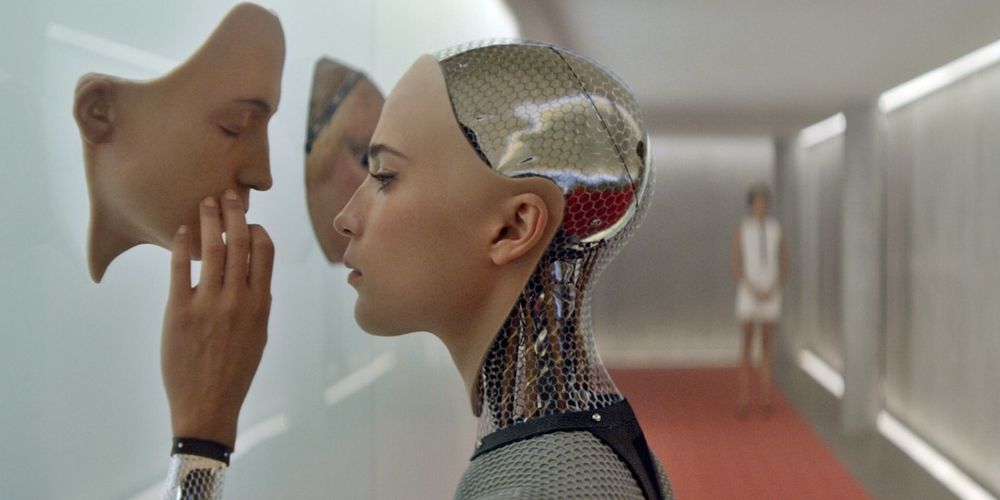
- 850 shares
- 1 year ago
Chatbots present a conversational interface to an application that attempts to mimic human interaction. Early chatbots used basic pattern matching to understand and respond to textual input. Machine learning has given way to Artificial Intelligence (AI), which uses deep learning and large language models. This is the basis of popular AI chatbots like OpenAI's ChatGPT and Google's Gemini.
While the history of chatbots starts in the 1960s, the original idea of "chatting" with a computer is the basis of the Turing Test. The test was published in 1950 by Alan Turing as part of his paper "Computing Machinery and Intelligence". It had the simple premise that if a text-based conversation with a computer is indistinguishable from that of a human, the computer has passed the test.
A chatbot called Eliza was one of the earliest examples. It was written by Joseph Weizenbaum in 1964. Eliza mimicked a psychotherapist and gave the appearance of understanding. Parry was another early example, designed by Kenneth Colby in 1972. It crudely modelled a paranoid schizophrenic and used a rule-based system to simulate human emotions.
Eliza Chatbot by Joseph Weizenbaum in 1966
Public Domain
Learn more about Eliza in this clip of Elaine Anzaldo, a Conversation Designer at Meta.
In 1972, the chatbots Eliza (simulating a psychiatrist) and Parry (simulating a schizophrenic) were connected at an international computer conference. A nonsensical conversation ensued, the opening of which is shown below. (It was recorded by Vint Cerf, one of the "fathers" of the Internet.)
The chatbots Parry and Eliza talking together in 1972. (Eliza is the doctor.)
Public Domain
In 1988, Jabberwacky was the first chatbot to use artificial intelligence, learning from previous conversations. Alice followed in 1995. She was inspired by Eliza and used pattern matching but made no attempt to understand the conversation as a whole. However, Alice was latter improved using a language created for AI called Aiml (AI Markup Language). This allowed the production of more human-like responses and resulted in her creator, Richard Wallace, winning the Loebner Prize Competition in 2000 and 2001.
The early 2000s saw a profusion of chatbots using AI. Chatbots from 2010 onwards tended to use the AI technique, natural language processing:
2001 Smarter Child (Active Buddy): A web-based chatbot on AOL and Windows Live Messenger, based on Microsoft technology. It could provide answers to practical questions by accessing sports, entertainment, news and weather databases.
2005 Mitsuku/Kuki (Steve Worswick): Mitsuku was designed as a general chatbot, using natural language processing. She has won the Leobner Prize (for seeming human) five times: in 2013 and each year from 2016 to 2019. Since 2019 she has been known as Kuki. Both Mitsuku and Kuki take on the persona of an 18-year-old Japanese student who speaks in English. They are implemented using Aiml and borrowed code from an earlier chatbot, Alice.
2010 Siri (Apple): The birth of the now ubiquitous personal assistant. At the time, Apple patented the idea of conversing with a chatbot through messages.
2012 Google Now/Google Assistant (Google): Originally conceived to provide location and time-specific on smartphones, Google Now was expanded to Google Assistant around 2017. Both could respond to voice requests.
2014 Alexa (Amazon): Alexa is the voice assistant built into Amazon Echo smart speakers. It is supported as a voice service on several platforms including Android, iOS, Linux, Windows and Wear. It is supported by a variety of entertainment devices and can also be used in cars through Amazon Echo Auto. Alexa uses natural language processing (NLP) but has limited ability compared with modern chatbots. In 2023 Amazon announced the incorporation of a large language model, similar to that used by ChatGPT, for example.
2014 Cortana (Microsoft): Cortana was a voice-based assistant that Microsoft saw as a key ingredient of future operating systems on Windows Phone and PC. It was named after an AI character in Microsoft's later Halo video games. It was withdrawn from phone platforms starting in 2020. It was officially deprecated in the Windows OS in 2023. Much of the vision that Microsoft had for integrating AI into operating systems was shifted to Microsoft Copilot chatbot.
2022 ChatGPT (OpenAI): GPT stands for generative pre-trained transformer. It uses a large language model (LLM) to build an "understanding" of human speech and knowledge, primarily from Internet sources. It uses the model to generate responses to questions. In more recent implementations, ChatGPT is also used to process and generate audio, video and still images. ChatGPT-4 was released in March 2023. The trained model has grown substantially in each release, as reflected by the number of parameters used in the underlying neural network:
GPT-1: 0.1 billion
GPT-2: 1.5 billion
GPT-3: 175 billion
GPT-4: 1,760 billion (1.76 trillion)
2023 Bard/Gemini (Google): Bard was launched in early 2023 in response to Microsoft's planned announcement to incorporate ChatGPT technology in its Bing search engine. (Microsoft is one of OpenAI's largest shareholders.) However, Bard met a lackluster response from industry, partly due to errors revealed during an early public demonstration of the technology. Google's response was to upgrade Bard to use a larger language model. Bard was relaunched as Gemini later in 2023.
2023 Bing Chat/Copilot (Micrososft): Bing Chat was introduced as a feature of the Bing search engine and Edge web browser. Microsoft's goal was to replace the Cortana chatbot feature built into its Windows operating system with a more powerful AI built on a Large Language Model. This was made possible by Microsoft's investment in, and collaboration with, OpenAI. Consequently, Bing Chat was based on ChatGPT-4. At the same time, Microsoft decided to rename Bing Chat as Copilot. It was built into Windows 11 during 2023 but was also available through the Edge browser. In 2024, Microsoft announced the integration of ChatGPT-4o into Copilot.
Chatbots come in a variety of shapes and sizes, depending on their purpose, abilities and interface:
Types of Chatbots (described below)
© Interaction Design Foundation, CC BY-SA 4.0
Before graphical user interfaces (GUIs) became the most common mode of interaction with computer systems, tree-like menu structures were often used. They provided a limited set of options at a point on a tree. A user's response would determine which branch of the tree was visited next. Menu systems were much easier to use than command-line systems and like GUIs, relied on recognition rather than recall.
Early menu-based chatbots could be implemented using purely text. The chatbot would display a list of numbered menu items. The user would type a number to choose the next action. (Alphabetic labels were also sometimes used.)
The screenshots below show a simple menu-based chatbot that might be used in a text-based environment like WhatsApp.
NumMenu Bot, an example of a menu-based chatbot.
https://github.com/DerXter/NumMenu-Bot
Menu-based chatbots are also possible within a GUI. Users click or tap on the questions themselves or buttons placed alongside.
Rules-based chatbots share some of the limitations of menu-based systems. A set of rules are typically written by developers for a narrow problem domain. Interaction is based on keyword detection, typically following a question and answer dialogue. Rules-based chatbots are very quick and require few resources, but they are unable to address topics outside of their defined domain. They do not learn from interactions and depend largely on provided content.
AI-powered chatbots use machine learning and natural language processing (NLP) to understand a wide range of conversational constructs and content. As a result, AI-based chatbots learn from interactions and can be trained on a broad range of subject areas. AI chatbots generally make use of deep neural networks but do not necessarily use the large language models found in general-purpose chatbots like OpenAI's ChatGPT. Nevertheless, AI chatbots can engage in very convincing, naturalistic, conversations.
Voice chatbots allow speech to be used for interaction. Interactive voice response (IVR) is a basic form of voice chatbot, but like rules-based and menu systems, they are usually limited to specific problem domains and a small set of keywords. With advances in AI, voice chatbots can engage in less structured conversations and are not as limited in terms of the breadth of subject matter that can be addressed.
Generative AI chatbots extend the deep learning enabled by neural networks to a range of media beyond simple text: images, audio and video. They are trained on large bodies of material. This enables them to create or modify media in response to requests from users. Generative AIs are the subject of much attention and improvement. They can create realistic output, including high-resolution video containing human and animal forms.
Opera's Aria AI-based chatbot describing what a chatbot is.
Public Domain
A chatbot is a computer program that can simulate human conversations using text or voice. Chatbots can interact with users through various platforms, such as websites, mobile apps, social media, messaging apps, and voice assistants. Chatbots can provide information, answer questions, perform tasks, or entertain users.
There are two main types of chatbots: rule-based and AI-based. Rule-based chatbots use predefined rules and decision trees to generate responses based on keywords and patterns. AI-based chatbots use machine learning and natural language processing to learn from user input and generate more personalized and context-aware responses.
Chatbots work by following a basic process:
First, the chatbot receives the user’s input, which can be text or speech.
Second, the chatbot analyzes the user’s input and extracts the intent and entities. The intent is the goal or purpose of the user’s input, such as booking a flight, ordering a pizza, or asking for the weather. The entities are the specific details or parameters related to the intent, such as the destination, the toppings, or the date.
Third, the chatbot generates a response based on the intent and entities. The response can be a simple text or speech output or a complex action that involves accessing other services or applications through APIs. The response can include follow-up questions or suggestions to keep the conversation going.
Fourth, the chatbot sends the response to the user and waits for the next input.
Chatbots have many benefits for both businesses and users, such as:
Chatbots can improve customer service and satisfaction by providing 24/7 support, instant answers, and personalized solutions.
Chatbots can increase sales and conversions by engaging customers, recommending products, and facilitating transactions.
Chatbots can reduce costs and save time by automating repetitive tasks, streamlining workflows, and optimizing resources.
Chatbots can enhance user experience and loyalty by creating interactive and immersive interactions, offering feedback and rewards, and learning from user preferences.
There are many examples of chatbots in various industries and domains, such as:
Customer service chatbots: These chatbots help customers with common queries, issues, or complaints. For example, Sephora uses a chatbot on Facebook Messenger to provide beauty tips, product recommendations, and booking services.
E-commerce chatbots: These chatbots help customers with shopping online. For example, H&M uses a chatbot on Kik to offer style advice, outfit suggestions, and purchase options.
Healthcare chatbots: These chatbots help patients with health-related questions or concerns. For example, Ada is a chatbot app that helps users diagnose their symptoms, find possible causes, and get appropriate guidance.
Education chatbots: These chatbots help students with learning or studying. For example, Duolingo is a chatbot app that helps users learn new languages through interactive conversations.
Entertainment chatbots: These chatbots help users with fun or leisure activities. For example, [Replika] is a chatbot app that helps users create their own personal AI companion to talk to anytime.
An AI chatbot is a chatbot that uses artificial intelligence (AI) technologies, such as machine learning and natural language processing, to understand the user’s questions and generate responses. AI chatbots can provide more accurate, relevant, and personalized answers than traditional chatbots that rely on predefined scripts or keywords.
Some examples of AI chatbots are:
IBM watsonx Assistant: A chatbot platform that allows businesses to create and deploy conversational AI solutions across various channels, such as websites, mobile apps, voice assistants, and messaging platforms. IBM watsonx Assistant uses natural language understanding (NLU) to discern the meaning and intent of user input and natural language generation (NLG) to produce natural and engaging responses.
Drift: A chatbot software that helps businesses generate and qualify leads, book meetings, and provide customer support. Drift uses conversational AI to create personalized and human-like conversations with website visitors, and integrates with various tools such as email, CRM, and calendar
Airdroid: A chatbot development platform that enables developers to build and deploy AI chatbots for various use cases, such as e-commerce, education, healthcare, and entertainment. Airdroid uses NLP and machine learning to create chatbots that can understand natural language, handle multiple intents, and provide contextual responses
Do you want to improve your UX / UI Design skills? Join us now
You earned your gift with a perfect score! Let us send it to you.
We've emailed your gift to name@email.com.
Do you want to improve your UX / UI Design skills? Join us now
Here's the entire UX literature on Chatbots by the Interaction Design Foundation, collated in one place:
Take a deep dive into Chatbots with our course AI for Designers .
In an era where technology is rapidly reshaping the way we interact with the world, understanding the intricacies of AI is not just a skill, but a necessity for designers. The AI for Designers course delves into the heart of this game-changing field, empowering you to navigate the complexities of designing in the age of AI. Why is this knowledge vital? AI is not just a tool; it's a paradigm shift, revolutionizing the design landscape. As a designer, make sure that you not only keep pace with the ever-evolving tech landscape but also lead the way in creating user experiences that are intuitive, intelligent, and ethical.
AI for Designers is taught by Ioana Teleanu, a seasoned AI Product Designer and Design Educator who has established a community of over 250,000 UX enthusiasts through her social channel UX Goodies. She imparts her extensive expertise to this course from her experience at renowned companies like UiPath and ING Bank, and now works on pioneering AI projects at Miro.
In this course, you’ll explore how to work with AI in harmony and incorporate it into your design process to elevate your career to new heights. Welcome to a course that doesn’t just teach design; it shapes the future of design innovation.
In lesson 1, you’ll explore AI's significance, understand key terms like Machine Learning, Deep Learning, and Generative AI, discover AI's impact on design, and master the art of creating effective text prompts for design.
In lesson 2, you’ll learn how to enhance your design workflow using AI tools for UX research, including market analysis, persona interviews, and data processing. You’ll dive into problem-solving with AI, mastering problem definition and production ideation.
In lesson 3, you’ll discover how to incorporate AI tools for prototyping, wireframing, visual design, and UX writing into your design process. You’ll learn how AI can assist to evaluate your designs and automate tasks, and ensure your product is launch-ready.
In lesson 4, you’ll explore the designer's role in AI-driven solutions, how to address challenges, analyze concerns, and deliver ethical solutions for real-world design applications.
Throughout the course, you'll receive practical tips for real-life projects. In the Build Your Portfolio exercises, you’ll practice how to integrate AI tools into your workflow and design for AI products, enabling you to create a compelling portfolio case study to attract potential employers or collaborators.
We believe in Open Access and the democratization of knowledge. Unfortunately, world-class educational materials such as this page are normally hidden behind paywalls or in expensive textbooks.
If you want this to change, , link to us, or join us to help us democratize design knowledge!