What to Test
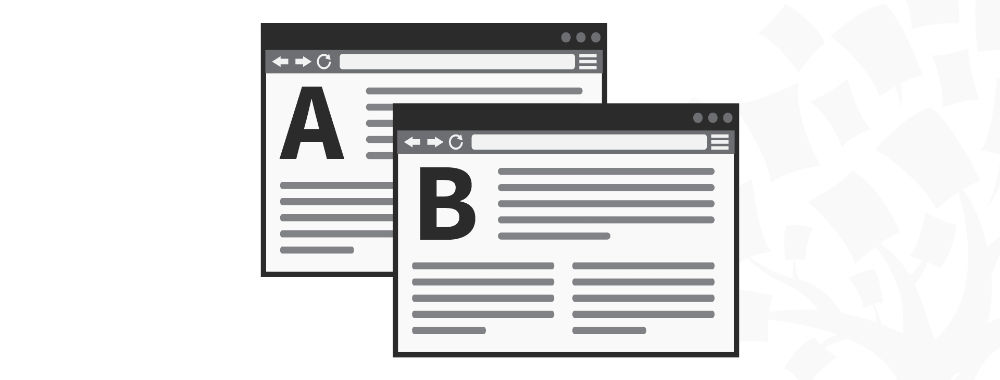
- 402 shares
- 3 years ago
A/B testing, or split testing, is a quantitative user research method. In A/B testing, researchers show different users two versions of the same design to identify which one performs better. The A refers to the original design, while the B refers to the variation of the A design.
A/B testing has applications in many fields, like marketing, social media and retail. However, user researchers and designers primarily use it to test website and application designs.
Researchers and designers use A/B testing to test individual page elements or minor layout variations. They keep everything else on the page the same except the aspect they want to test. This way, they know that any difference in results comes from the variation alone.
A/B testing is quick, easy, and cheap. Designers and researchers choose this method to test slight differences in their designs.
© Interaction Design Foundation, CC BY-SA 4.0
For example, the online streaming platform, Netflix, used A/B/n testing to find which call to action button resulted in more sign-ups. A/B/n testing extends A/B testing by incorporating more than one design variant.
Netflix split site visitors between these four design alternatives. They kept everything the same except the button to ensure their results would be reliable. Once the test was complete, they implemented the phrase “Get Started,” as it resulted in significantly more sign-ups than the other three designs.
© Netflix, Fair use
A/B testing typically measures the difference in conversion rate between two designs. The conversion rate is the percentage of users who complete a desired action. Some example actions include:
Add item to cart.
Donate money to charity.
Sign up for a newsletter.
Click a specific item in a menu.
Other metrics that A/B testing can measure include:
The time a user spends on a page or site.
The percentage of users who leave a site after viewing only one page (the bounce rate).
A/B testing is limited in what it can measure. However, the variables that researchers can A/B test are almost limitless. Researchers change one variable between design variants and compare the metrics. Here are some examples of variables:
Example | Variable 1 | Variable 2 | Variable 3 |
Style (horizontal vs. vertical) | Icons vs. text | Placement (top, bottom, side) | |
Number of columns | Above-the-fold content | Sidebar presence and position | |
Buttons | Shape and size | Text (“Add to Cart” vs. “Buy Now”) | |
Forms | Number of fields | Field types (dropdowns, text input) | Layout and ordering of fields |
Font styles and sizes | Text color and contrast | Line spacing and text alignment | |
Images and videos | Placement and size | Static vs. carousel | Thumbnails vs. full-size images |
Overall color theme | Contrast ratios | Button and link colors | |
CTA (call-to-action) elements | Placement on the page | Wording and urgency | Design and visibility |
Content strategy | Headlines and subheadings | Length and style of copy | Use of bullet points vs. paragraphs |
Alt text for images | Keyboard navigation | Screen reader friendliness | |
Error messages | Wording and tone | Instructions for resolution | Sound effects |
Search box placement and design | Search algorithms | Filters and sorting options | |
Pop-ups and modals | Timing and frequency | Offer types (newsletter sign-up, discount codes) | Exit-intent vs. timed display |
Email capture forms | Placement and timing | Incentives (discounts, ebooks) | Design elements |
Push notifications | Timing and frequency | Content and call to action | Sound effects |
Meta titles and descriptions | Headings structure (H1, H2, H3) | Keyword placement | |
Pricing strategies | Pricing display ($10 vs. $9.99) | Subscription models vs. one-time purchases | Anchor Pricing (display a higher priced item next to the main product) |
Sales and discounts | Types of discounts (percentage off vs. buy one get one) | Placement of sale information | Original price crossed out vs. savings amount highlighted |
“Testing leads to failure, and failure leads to understanding.”
—Burt Rutan
User researchers and designers use testing to make data-driven design decisions and optimize their products' user experience (UX). A/B testing is a highly effective user research method that is:
Cost-effective. Researchers can implement A/B testing with live users following deployment. This approach eliminates the need for expensive pre-launch testing environments. For example, a product manager wants to test two landing pages to see which results in more sign-ups. They split the website's traffic between the two versions. The A/B test gives them valuable data without a significant increase in costs.
Efficient. A/B testing provides rapid results, especially for products with substantial user bases. Sometimes, two weeks of testing is enough to collect actionable data.
Straightforward. Analytics tools provide researchers with clear insights into which design variant performs best. Researchers evaluate outcomes based on predefined metrics, like conversion rates. For instance, a researcher tests two call to action buttons. Analytics reveal the variant that leads to higher conversions. These results provide a clear directive for researchers on which element enhances the user experience.
In this video, William Hudson explains how to fit quantitative research into the project lifecycle:
A/B testing is unsuitable for assessing the qualitative aspects of user experience. Qualitative aspects include:
Satisfaction.
Comprehension.
Given this, researchers must know what they want to achieve before testing.
For instance, if a researcher relies solely on A/B testing to enhance user satisfaction, it would not provide the insights needed. A/B testing can show users spend more time on a page but cannot explain why users feel more engaged.
When researchers want to understand the 'why' behind user behaviors, they use other research methods. More suitable methods include user interviews, usability testing and surveys. These methods complement the quantitative data from A/B testing.
Before a researcher can conduct an A/B test, their website or app must be fully functional. Test results will be unreliable for unfinished products.
For instance, a designer wants to test a product page for a mobile phone case. The page has:
A dropdown menu to choose the case color.
Product photos that change when the user selects a different color.
An “add to basket” button.
The designer creates two designs with different "add to basket" button placements. However, the drop-down list is not functioning correctly. When the user chooses a case color, the product photos change to the wrong color. If users become frustrated, the button's placement will unlikely affect their decision to add to the basket. Any results from the test will be unreliable.
Designers and researchers use A/B testing in the late stages of the development cycle or after deployment. A/B tests need stable, well-designed environments to function correctly.
© Interaction Design Foundation, CC BY-SA 4.0
Also, the number of users tested must be significant enough to see actionable results. Researchers can conduct longer tests for smaller audiences to reach the required sample size. A/B/n testing requires a larger pool of users than A/B testing. More design alternatives mean more participant groups.
A/B sample size calculators help researchers specify a target sample size based on their website’s existing analytics.
Before user researchers conduct testing, they define the questions they want to answer. An example of a bad question is, “Will better product photos reduce the number of customer service queries?” Researchers cannot effectively A/B test this. Many channels to customer service exist, not just product pages.
In this scenario, a good question is, “Will different product photos improve conversions?” Researchers split their users between two different designs, each with different product photos. If significantly more users purchase the product via design B, researchers can be confident:
Users are ordering more.
They are less likely to go to customer service.
Another bad example is, “Will shortening the sign-up process improve user satisfaction?” Satisfaction is challenging to measure with A/B testing, and many ways exist to shorten a sign-up process. The question must be more specific and design-related. For example, “Which design, A or B, leads to more sign-ups?”
Once researchers and designers are confident their product is sound and has enough users, they follow a three-part process for A/B testing.
Researchers do not need to complete these steps each time they A/B test. However, for first-time A/B testing, these steps are crucial:
Identify key stakeholders. Discover who needs to agree or give resources for the testing. Requirements include getting:
Funding and permission from managers.
Access to existing A/B testing tools and data.
While A/B testing is inexpensive, managers must still approve its use. Marketing or development teams may hold the keys to existing analytics implementations. Finally, design and research colleagues may need to create alternative designs and run the test.
© Interaction Design Foundation, CC BY-SA 4.0
Convince stakeholders of A/B testing's value. It's crucial everyone involved understands why A/B testing is useful. This understanding is critical in scenarios where stakeholders might not be familiar with UX design. Clear examples, like stories of past successes, show stakeholders how A/B testing has helped other projects or companies.
Set up the necessary tools. Choose and set up the software for web analytics and A/B testing. Find the right tools that fit the project's needs and set them up.
Once researchers have the required access, permissions and funding, they prepare for the test:
Define research questions. Decide the questions that need answering. For example, “Will changing the button color of a call to action result in more clicks?”
Design the alternatives. Next, create the designs you will test against each other. Make sure these designs are as perfect as possible. For shorter tests, some flaws are acceptable.
Select your user group(s) (optional). Most A/B testing and analytics software allows you to filter results by user group. For this reason, testing specific groups is not always necessary, as you can specify this later. However, if the software doesn’t allow this, you should define this before testing.
Plan your schedule. Finally, decide on a timeline for your test that includes when you'll start, how long it will run and when you'll check on the results. A clear schedule helps manage the test without wasting time or resources.
Once the testing period has finished, researchers view the results and decide their next steps:
Check if the results are reliable. Look at the analytics to see if the differences are significant enough. Minor differences between the performance of designs A and B may be chance. Researchers use methods like chi-square tests to determine whether the results are significant.
If the results are unclear, change the designs and rerun the test, or run the test longer to get more data. These solutions help make sure the next test gives more apparent answers.
If the results are clear, implement the better version.
Keep improving. Researchers don’t only A/B test once; it's an ongoing process. Findings inform and inspire future tests.
Researchers interpret A/B test results to make informed decisions about design choices. A/B testing results are typically straightforward (e.g., which design resulted in more conversions). However, researchers must determine if the results are statistically significant.
Researchers use the chi-square test, a fundamental statistical tool. Chi-square tests play a pivotal role in A/B testing. They reveal whether observed results are statistically significant or chance findings.
Chi-square test results are easy to interpret. If the test indicates a significant difference, researchers can be confident which design is best. For example, a researcher tests two web page versions to increase conversions:
Version A gets 5000 visitors with 100 sign-ups.
Version B gets 5000 visitors with 150 sign-ups.
The researcher analyzes these results using an online chi-square calculator:
They enter each design's successes (sign-ups) and failures (no sign-ups).
They set the significance level at 0.05 (or 5%—the most typical level).
The chi-square test provides a P-value of 0.001362, which is lower than the significance level. Any P-level value under 0.05 is considered statistically significant, while any value over is considered chance.
In this scenario, the researcher is confident their results are statistically significant. They can make design decisions based on these results.
The chi-square test determines if A/B test results are statistically significant. In this example, the difference between conversions may seem small compared to the total users. However, the P-value (the output of the chi-square test) is much lower than the significance level—it is statistically significant. Chi-square tests give researchers and designers the confidence to make data-driven decisions.
© Interaction Design Foundation, CC BY-SA 4.0
Researchers follow these best practices to run A/B tests:
Understand the platform well. Researchers should be familiar with the product before conducting A/B testing. A lack of knowledge leads to unreliable and unuseful results within the context of the platform.
Know the users. Researchers must understand who their users are and what they need from the product. This knowledge is available from existing user research, data and findings.
Choose what to test wisely. Researchers focus on the parts of their site that affect their users the most. For example, an excellent place to start is with user complaints. Other sources, like heat maps and session recordings, provide researchers with test subjects.
Talk to stakeholders. Management and other departments might see problems or have ideas the design team is unaware of.
Set clear goals. Researchers know what they want to achieve with A/B testing. They set measurable goals to guide testing and ensure relevance and focus.
Small changes, big impact. Design changes should be small. Significant changes and overhauls can confuse and upset users. Researchers focus on minor tweaks that make substantial differences.
Use segmentation. Segmentation is helpful after a completed test to review different user groups. Researchers compare demographics and segments like mobile and desktop website visitors.
A/B testing is typically straightforward and inexpensive. However, researchers must be aware of its limitations and potential stumbling blocks.
Requires a large user base. A/B testing only provides trustworthy results with a sufficient user pool. Without enough people, it might take longer to get results, or the findings might not be reliable.
Outside factors can influence results. External factors like seasonal changes and new trends can negatively affect results. For example, a retailer runs an A/B test on their website during the holiday season to determine the effectiveness of new product photos. However, the increased traffic and buying intent during the holiday season inflates the success of the images. In a regular season, the photos would likely not perform as well.
Focuses on short-term goals. A/B testing typically focuses on immediate results, like how many people click on a button. Long-term goals like customer happiness and brand loyalty are difficult to assess. For instance, a news website runs an A/B test comparing two headline styles to see which generates more clicks. One style leads to a higher click-through rate but relies on clickbait titles that may erode trust over time.
Ethical Concerns. Some tests significantly change what users experience or how products handle their privacy. In these scenarios, researchers must consider ethical practices. For example, an e-commerce site tests an alternative checkout process that adds a last-minute upsell offer. The offer could frustrate users who want to complete their purchases quickly.
Researchers use multivariate testing to test multiple variables between two or more designs. This method is more complex than A/B testing. Researchers may choose multivariate testing over A/B testing for the following reasons:
Complex interactions. It is suitable for examining how multiple variables interact with one another. Multivariate testing can provide insights into more complex user behaviors.
Comprehensive analysis. It allows for a more detailed analysis of how different elements of a page or product work together. This detail can lead to more nuanced improvements.
Optimizes multiple variables simultaneously. It is ideal for optimizing several aspects of a user experience at once. This optimization can lead to significant improvements in performance.
For example, during the 2008 US presidential election, the Obama campaign used multivariate testing to optimize newsletter sign-ups. They tested different combinations of their homepage media (an image or a video) and the call to action button. The team preferred one of the videos. However, testing revealed that an image performed better. This example highlights the importance of user testing and user-centered design.
The Obama campaign tested 4 CTA and six media variations (three images and three videos). They found that design option 11 (right image) had 40.6% more signups than the original page (left image). They implemented the more successful design, translating to approximately 2,880,000 additional signups throughout the campaign. These extra signups resulted in an additional USD 60,000,000 in donations.
© Optimizely, Fair use
Researchers may choose A/B testing over multivariate testing for the following reasons:
Simplicity and focus. It is more straightforward to set up and analyze, comparing two versions of a single variable to see which performs better.
Quick to implement. It allows for rapid testing and implementation of changes. This efficiency is ideal for iterative design improvements.
Requires less traffic. It achieves statistically significant results with less traffic. This benefits sites with smaller user bases.
Clear insights. Offers straightforward insights, making it easier to make informed decisions.
Researchers employ many types of research methods. A/B testing is a powerful tool, but other methods can be more appropriate depending on the situation.
© Interaction Design Foundation, CC BY-SA 4.0
User researchers understand various user research methods. While A/B testing is helpful in many situations, here are four alternatives and why researchers might choose them instead.
Paper prototyping is an early-stage method researchers use for quick, hands-on idea testing. Unlike A/B testing, paper prototyping is about ideation and immediate reactions. Researchers use this method to generate quick feedback on basic design concepts. Paper prototyping happens before the costly development phase. This approach helps researchers quickly identify user preferences and usability hurdles.
Card sorting dives deep into how users mentally organize information. This method offers insights that are sometimes not revealed in A/B testing. Researchers employ card sorting to structure or restructure a product's information architecture. Users group content into categories and reveal patterns that guide information organization. This method ensures the final structure aligns with user expectations.
Tree testing focuses on evaluating the navigational structure of a site. Designers and researchers use this method to refine an existing navigation. Tree testing can also confirm a new structure's usability. This method strips away the visual design elements and focuses on how easily users can find information. Researchers choose this targeted approach over A/B testing to identify navigational issues.
First-click testing assesses a web page layout's immediate clarity and key actions. Researchers use this method to understand if users can quickly determine where to click to complete their goals. A/B testing does not always reveal this information. First-click testing offers precise feedback on the effectiveness of the initial user interaction.
Learn more about A/B testing and other practical quantitative research methods in our course, Data-Driven Design: Quantitative Research for UX.
Jakob Nielsen discusses how A/B testing often puts the focus on short-term improvements.
Find out how and why Netflix implements A/B testing across their platform.
Learn how to Define Stronger A/B Test Variations Through UX Research with the Nielsen Norman Group.
Discover how the 2008 Obama presidential campaign used multivariate testing.
Watch our Master Class with Zoltan Kollin, Design Principal at IBM, for further insights into A/B testing
A large portion of A/B tests do not show a clear improvement. Various factors can contribute to this high failure; for example:
Small sample sizes.
Short testing periods.
Minor changes that don't significantly impact user behavior.
However, these "failures" are invaluable learning opportunities. They provide insights into user preferences and behavior. These insights help researchers refine their hypotheses and approaches for future tests.
To increase the success rate of A/B tests, researchers ensure they have:
A clear hypothesis.
A sufficiently large sample size.
A significant enough variation between the tested versions.
A sufficient test duration to account for variability in user behavior over time.
Don Norman, founding director - Design Lab, University of California, explains how every failure is a learning opportunity:
David M. Kelley by Jonathan Chen (CC BY 2.0)
https://www.flickr.com/photos/wikichen/9375796736/
To conduct A/B testing, researchers can use various tools to set up design alternatives and measure outcomes. Popular tools include:
Google Optimize offers seamless integration with Google Analytics (GA). This integration allows researchers to use their existing GA goals as test objectives. Researchers can easily visualize how their experiments impact user behavior.
Optimizely is a powerful tool that allows extensive experimentation. Researchers can use this platform across websites, mobile apps and connected devices. Optimizely makes it easy for researchers to create and modify experiments without writing code.
VWO (Visual Website Optimizer) provides a suite of tools, including A/B testing, multivariate testing, and split URL testing. VWO’s interface is designed for marketers, making it accessible for those with limited technical skills.
Unbounce is best for testing landing pages. Its drag-and-drop editor enables researchers to create and test landing pages without developer resources.
Adobe Target is part of the Adobe Experience Cloud. This tool suits businesses looking for deep integration with other Adobe products.
These tools allow researchers to make data-driven decisions that enhance user experience. However, success in A/B testing comes from more than just tools. Clear objectives, appropriate metrics and iteration based on findings lead to profitable outcomes.
William Hudson, CEO of Syntagm, UX Expert and Author, explains how researchers and designers use analytics in UX design:
Author: Stewart Cheifet. Appearance time: 0:22 - 0:24. Copyright license and terms: CC / Fair Use. Modified: Yes. Link: https://archive.org/details/CC1218greatestgames
If both versions in an A/B test perform similarly, it suggests the changes tested did not significantly impact user behavior. This outcome can have several reasons:
Insensitivity to changes. The tested element might not influence user decisions.
Need for more significant changes. Consider testing more noticeable variations.
Well-optimized existing design. The current design effectively meets user needs.
Inconclusive results. The test duration was too short, or the sample size too small.
If A/B tests remain inconclusive, researchers should use different methods to explore more profound insights. Methods include surveys, interviews and usability testing.
Develop a foundational understanding of user research with our course, User Research: Methods and Best Practices.
A/B testing results can mislead due to:
Methodological errors. Unclear questions, biased groups and test groups that are too small.
Incorrect data interpretation. Confusion about significance, not seeing random changes and bias towards expected outcomes.
Overlooking factors. Time of year, market changes and technology updates.
Here's how researchers can mitigate these risks:
Test for statistical significance. Confirm if results are statistically significant or chance findings.
Control external factors. Isolate tests from external factors or account for them.
Run tests for adequate duration. Capture user behavior variations with sufficient test periods.
Avoid multiple changes. Test one design change at a time for clear outcomes.
Focus on user experience. Consider long-term user satisfaction and retention impacts.
Peer review. Ask colleagues to review findings for overlooked errors or biases.
Continuous testing. Refine understanding through ongoing testing and iteration.
This risk mitigation allows researchers and designers to make informed design decisions. Take our course, Data-Driven Design: Quantitative Research for UX, to learn how to run successful A/B and multivariate tests.
User consent is pivotal in A/B testing amidst growing privacy concerns and strict data protection laws like GDPR and CCPA. Here's why user consent matters:
Ethical consideration. Ask for user consent before data collection. This approach honors user privacy and autonomy.
Legal compliance. Explicit consent is often mandatory for data collection and processing. A/B testing data can sometimes personally identify users.
Trust building. Brands that communicate their data practices clearly and respect user choices often gain user trust.
Data quality. Consented participation typically comes from engaged and informed users. This type of user usually provides higher-quality data.
To weave user consent into A/B testing:
Clearly inform users. Clearly explain the A/B test's nature, the data to be collected, its use and the voluntary basis of their participation.
Offer an opt-out. Ensure an accessible opt-out option for users that acknowledges their privacy and choice rights.
Privacy by design. Embed privacy considerations into A/B testing frameworks from the outset. Focus on essential data collection and securing it properly.
Researchers incorporate user consent to align with legal requirements and strengthen user relationships. Learn more about credibility, one of the seven key factors of UX, in this video:
A few key differences exist between A/B testing for B2B (business-to-business) and B2C (business-to-consumer) products:
Decision-making process. B2B tests target multiple stakeholders in longer processes. B2C focuses on emotional triggers and immediate value for individual consumer decisions.
Sales cycle length. B2B's longer sales cycles require extended A/B testing durations. B2C's shorter cycles allow for rapid testing and iterations.
Content and messaging. B2B A/B testing emphasizes information clarity and return-on-investment (ROI) demonstration. B2C testing focuses on emotional appeal, usability, and instant gratification.
Conversion goals. B2B tests often aim at lead generation (e.g., form submissions and whitepaper downloads). B2C targets immediate sales or sign-ups.
User volume and data collection. B2C's more extensive user base facilitates richer data for A/B testing. B2B's niche markets may necessitate more extended tests or multivariate testing for significant data.
User behavior. B2B testing focuses on functionality and efficiency for business needs. B2C prioritizes design, ease of use and personal benefits.
Regulatory considerations. B2B faces stricter regulations affecting test content and data handling. B2C has more flexibility but must respect privacy laws.
Researchers must understand these differences to conduct A/B testing in each domain effectively.
While A/B testing is well known for optimizing website conversion rates and user experience, it is helpful in other areas:
Content strategy. A/B testing can inform what most engages your audience. Refine strategies by testing storytelling methods, article lengths and formats (videos vs. text).
Email design. Test newsletters to enhance open rates and engagement. Experiment with alternative layouts, imagery and interactive features to understand visual preferences.
Voice and tone. Tailor communication to your users effectively. Experiment with voice and tone of content and copy to uncover user preferences.
Error messages and microcopy. Test microcopy variations like error messages to guide users through errors or challenges.
Accessibility. Improve the effectiveness of accessibility features. For example, test the accessibility toolbar placement where users engage with it more.
Torrey Podmajersky, Author, Speaker and UX Writer at Google, explains her process for writing notifications, which includes A/B testing:
King, R., Churchill, E., & Tan, C. (2016). Designing with Data: Improving the User Experience with A/B Testing. O’Reilly.
This book explores the relationship between design practices and data science. King, Churchill and Tan advocate for data-driven A/B testing to refine user experiences. The book details the process for implementing A/B testing in design decisions, from minor tweaks to significant UX changes. It includes real-world examples to illustrate the approach.
Kohavi, R., Tang, D., & Xu, Y. (2022). Trustworthy Online Controlled Experiments: A Practical Guide to A/B Testing. Cambridge University Press.
This book compiles the expertise of leaders from Google, LinkedIn and Microsoft. It covers the design, execution and interpretation of A/B tests. Kohavi, Tang and Xu offer insights into practical applications and real-world examples. Applications include enhancing product features, efficiency and revenue.
Georgiev, G. (2019). Statistical Methods in Online A/B Testing: Statistics for Data-Driven Business Decisions and Risk Management in E-commerce. Independent.
This book focuses on statistical methods for A/B testing. It demystifies complex concepts, making them accessible to professionals with minimal mathematical background. Georgiev covers practical applications in business, risk management and decision-making through online experiments. This book elevates the reader's A/B testing practices in various digital contexts.
Do you want to improve your UX / UI Design skills? Join us now
You earned your gift with a perfect score! Let us send it to you.
We’ve emailed your gift to name@email.com.
Do you want to improve your UX / UI Design skills? Join us now
Here’s the entire UX literature on A/B Testing by the Interaction Design Foundation, collated in one place:
Take a deep dive into A/B Testing with our course Data-Driven Design: Quantitative Research for UX .
Quantitative research is about understanding user behavior at scale. In most cases the methods we’ll discuss are complementary to the qualitative approaches more commonly employed in user experience. In this course you’ll learn what quantitative methods have to offer and how they can help paint a broader picture of your users’ experience of the solutions you provide—typically websites and apps.
Since quantitative methods are focused on numerical results, we’ll also be covering statistical analysis at a basic level. You don’t need any prior knowledge or experience of statistics, and we won’t be threatening you with mathematical formulas. The approach here is very practical, and we’ll be relying instead on the numerous free tools available for analysis using some of the most common statistical methods.
In the “Build Your Portfolio: Research Data Project”, you’ll find a series of practical exercises that will give you first-hand experience of the methods we’ll cover. If you want to complete these optional exercises, you’ll create a series of case studies for your portfolio which you can show your future employer or freelance customers.
Your instructor is William Hudson. He’s been active in interactive software development for around 50 years and HCI/User Experience for 30. He has been primarily a freelance consultant but also an author, reviewer and instructor in software development and user-centered design.
You earn a verifiable and industry-trusted Course Certificate once you’ve completed the course. You can highlight it on your resume, your LinkedIn profile or your website.
We believe in Open Access and the democratization of knowledge. Unfortunately, world-class educational materials such as this page are normally hidden behind paywalls or in expensive textbooks.
If you want this to change, , link to us, or join us to help us democratize design knowledge!